Einstein Recipes: The most powerful personalization tool which uses the machine learning algorithms to promote personalized content to each customer in the form of recommendations on the website, emails and mobile which result in increase in sales/revenue and customer engagement.
Recipes are most often used in ecommerce websites to recommend relevant products and in B2B and Financial services for content recommendations.
The combination of the four components of a recipe helps in delivering powerful one to one recommendation.
- Ingredients: Ingredients are the base algorithms to configure a recipe.
- Co-occurrence algorithms: Uses an anchor item as the basis for their scoring.
- Machine Learning algorithms: Collaborative Filtering is used in the recommendations system
- Catalog -based algorithms: Catalog-based ingredients surface items from the catalog based on catalog-level information and catalog-wide user behaviour.
- Filters: Filters are applied to include or exclude specific variables and avoid common pitfalls.
- Boosters: Boosters pull affinity data from the user profile based on which the relevant recommendations get a boost.
- Variations: Variations are determined to force variation in recommendation results so the visitor will have a broad range of options.
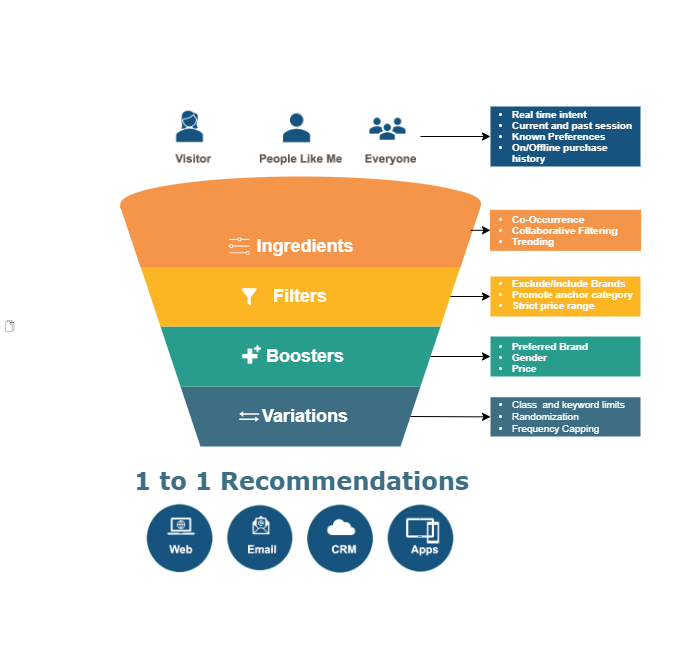
Outcome of a Recipe: The business user should have a good knowledge on using the recipes to get most of it by defining when and where to use a particular recipe with the specific powerful ingredients. Recipes can be tested and simulated for the test groups to see which is having more impact.
Einstein Recipe helps the visitor to view the most relevant products, recommendations and influence the decisions.
